
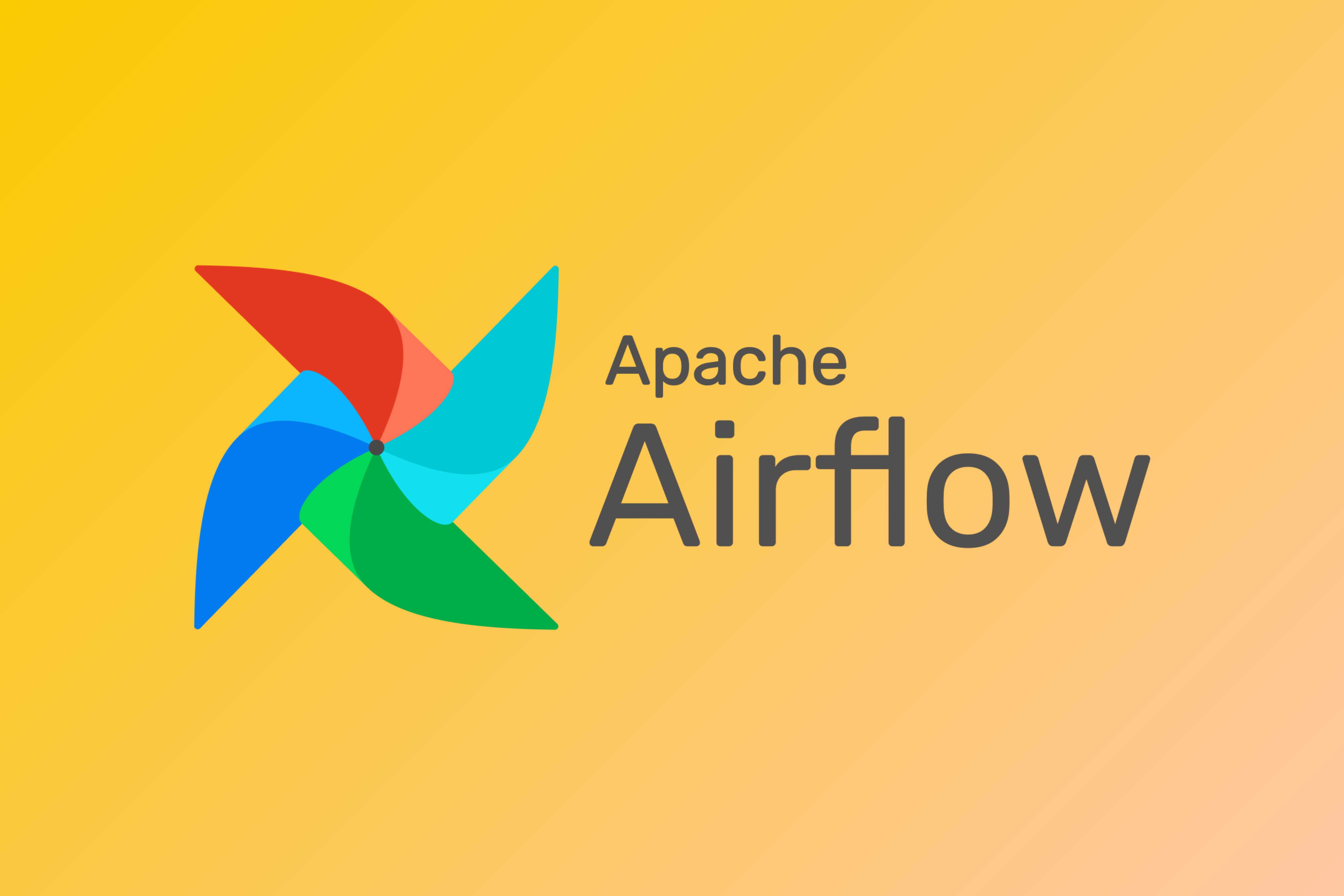
#AIRFLOW DAG LICENSE#
See the License for the # specific language governing permissions and limitations # under the License.
#AIRFLOW DAG SOFTWARE#
You may obtain a copy of the License at # Unless required by applicable law or agreed to in writing, # software distributed under the License is distributed on an # "AS IS" BASIS, WITHOUT WARRANTIES OR CONDITIONS OF ANY # KIND, either express or implied. The ASF licenses this file # to you under the Apache License, Version 2.0 (the # "License") you may not use this file except in compliance # with the License. See the NOTICE file # distributed with this work for additional information # regarding copyright ownership. # Licensed to the Apache Software Foundation (ASF) under one # or more contributor license agreements.
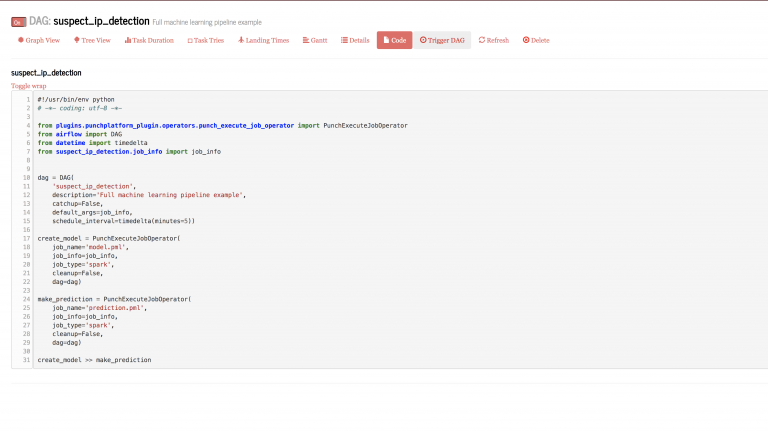
datetime ( 2022, 1, 1 ), schedule =, tags =, ) as dag : start = EmptyOperator ( task_id = "start", ) section_1 = SubDagOperator ( task_id = "section-1", subdag = subdag ( DAG_NAME, "section-1", dag. Defaults to """ get_ip = GetRequestOperator ( task_id = "get_ip", url = "" ) ( multiple_outputs = True ) def prepare_email ( raw_json : dict ) -> dict : external_ip = raw_json return, start_date = datetime. datetime ( 2021, 1, 1, tz = "UTC" ), catchup = False, tags =, ) def example_dag_decorator ( email : str = ): """ DAG to send server IP to email. Schedule interval put in place, the logical date is going to indicate the timeĪt which it marks the start of the data interval, where the DAG run’s startĭate would then be the logical date + scheduled ( schedule = None, start_date = pendulum. If you want to implement a DAG where number of Tasks (or Task Groups as of Airflow 2.6) can change based on the output/result of previous tasks, see Dynamic Task.

However, when the DAG is being automatically scheduled, with certain This document describes creation of DAGs that have a structure generated dynamically, but where the number of tasks in the DAG does not change between DAG Runs.

Logical is because of the abstract nature of it having multiple meanings,ĭepending on the context of the DAG run itself.įor example, if a DAG run is manually triggered by the user, its logical date would be theĭate and time of which the DAG run was triggered, and the value should be equal (formally known as execution date), which describes the intended time aĭAG run is scheduled or triggered. Manage the allocation of scarce resources. Ensures jobs are ordered correctly based on dependencies. Run’s start and end date, there is another date called logical date Airflow is a Workflow engine which means: Manage scheduling and running jobs and data pipelines. This period describes the time when the DAG actually ‘ran.’ Aside from the DAG Tasks specified inside a DAG are also instantiated intoĪ DAG run will have a start date when it starts, and end date when it ends. In much the same way a DAG instantiates into a DAG Run every time it’s run, Run will have one data interval covering a single day in that 3 month period,Īnd that data interval is all the tasks, operators and sensors inside the DAG Those DAG Runs will all have been started on the same actual day, but each DAG The previous 3 months of data-no problem, since Airflow can backfill the DAGĪnd run copies of it for every day in those previous 3 months, all at once. It’s been rewritten, and you want to run it on Same DAG, and each has a defined data interval, which identifies the period ofĪs an example of why this is useful, consider writing a DAG that processes aĭaily set of experimental data. If schedule is not enough to express the DAG’s schedule, see Timetables.įor more information on logical date, see Data Interval andĮvery time you run a DAG, you are creating a new instance of that DAG whichĪirflow calls a DAG Run. For more information on schedule values, see DAG Run.
